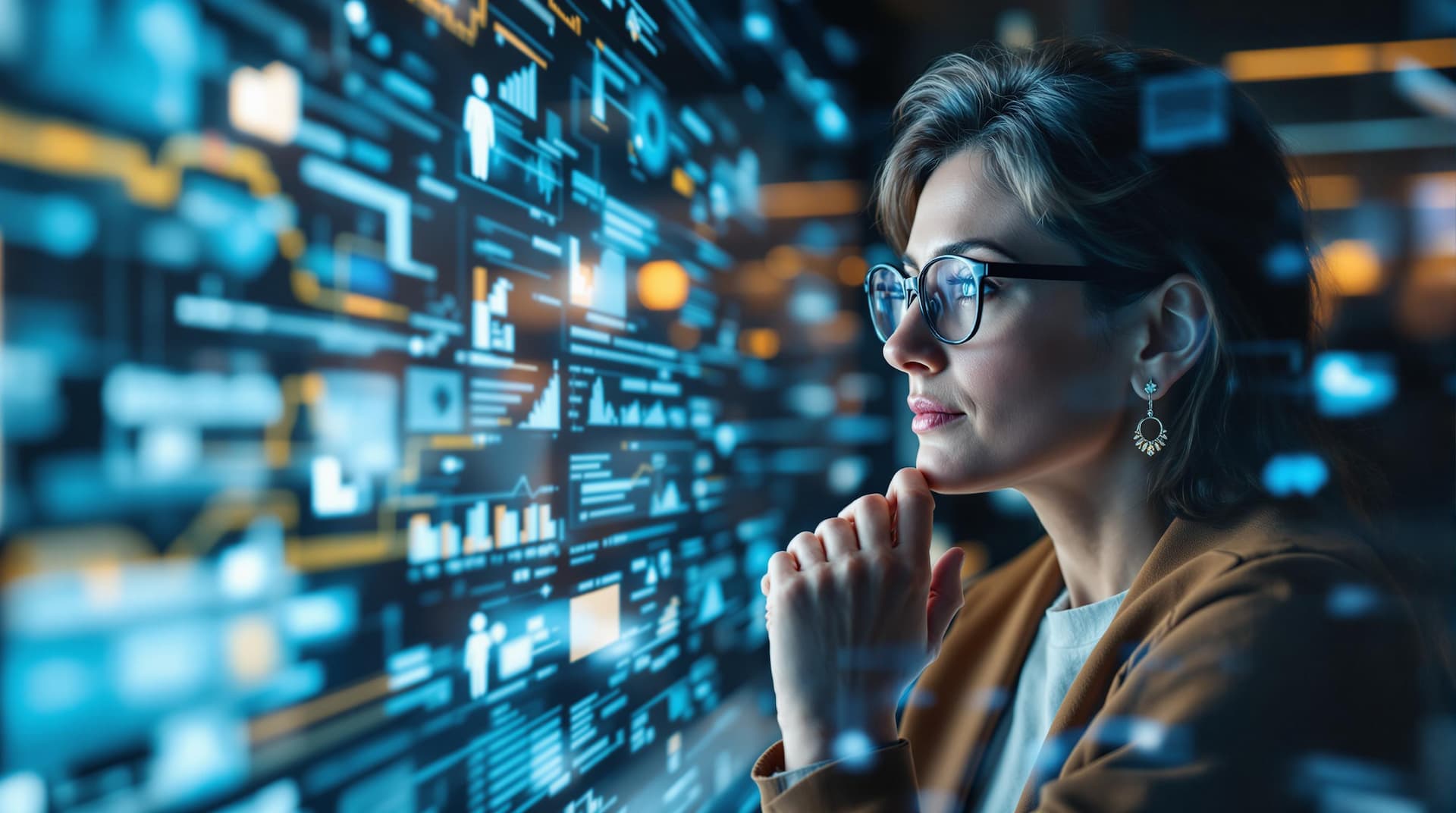
What Causes AI Detection False Positives
AI detection tools often mislabel human-written content as AI-generated, causing false positives. This happens due to algorithm limitations, complex writing styles, and specialized language. These errors can harm reputations, waste time, and unfairly target students or professionals. Here's what you need to know:
-
Main Causes:
- Outdated algorithms and limited training data.
- Complex sentence structures and technical jargon.
- Repetitive or formal writing styles.
-
Impacts:
- Academic integrity issues.
- Professional credibility risks.
- Wasted resources and time.
-
Solutions:
- Use multiple tools for cross-checking.
- Conduct manual reviews for flagged content.
- Improve AI models with diverse and updated data.
False positives remain a challenge, but combining technology with human oversight can improve accuracy and fairness.
Reasons for AI Detection False Positives
Limitations of Algorithms
AI detection tools often face challenges due to limited training data and outdated models. Research indicates that these algorithms struggle with diverse writing styles because they haven't been exposed to enough varied examples during their development.
This problem becomes worse as language evolves. Since detection tools rely on older models, they can lag behind changes in both human writing styles and AI-generated content. This creates a "detection gap", where newer, legitimate human writing patterns are mistakenly flagged as AI-generated . Such gaps raise concerns about fairness, as discussed earlier.
Complex Sentence Structures
Detection tools also struggle with complex sentence structures. When writers use sophisticated language or sentences with multiple dependent clauses, algorithms may incorrectly label the text as AI-generated. This issue is particularly common in academic writing, where intricate sentence patterns are standard .
Use of Specialized Language
Specialized vocabulary often leads to false positives. Here's how it plays out across different fields:
Field | Common Triggers |
---|---|
Academic Research | Technical jargon, standardized expressions |
Legal Documents | Legal terminology, formal phrasing |
Medical Writing | Clinical terms, procedural descriptions |
Technical Documentation | Industry-specific acronyms, technical details |
These errors can harm professional credibility, as noted earlier.
Formal or Repetitive Writing
Formal and repetitive writing styles, especially in academic or professional contexts, often resemble patterns detected in AI-generated content. For example, in spring 2023, Turnitin's testing showed that their AI detection software wrongly flagged parts of completely human-written academic essays as AI-generated . This reflects how consistent tone and structure can confuse detection tools, further complicating accurate evaluations.
Effects of False Positives in AI Detection
Impact on Academic Integrity
False positives in AI detection can unfairly target students with advanced writing styles, especially in technical fields. This issue disproportionately impacts high-performing students, international students, and technical writers, raising concerns about systemic bias. The implications extend beyond the classroom, potentially affecting professional opportunities.
"We cannot mitigate the risk of false positives completely given the nature of AI writing and analysis, so, it is important that educators use the AI score to start a meaningful and impactful dialogue with their students in such instances." - Annie Chechitelli, Chief Product Officer, Turnitin
Risks in Professional Settings
The stakes are even higher in professional environments, where credibility and reputation are crucial. When AI detection tools mistakenly flag legitimate work, the consequences can be severe:
Sector | Impact |
---|---|
Publishing | Delays in releases |
Marketing | Loss of clients |
Technical Fields | Damage to credibility |
This situation may push professionals to oversimplify their writing to avoid being flagged, potentially lowering the quality of their output.
Time and Resource Wastage
Addressing false positives consumes valuable time and resources. Investigations often require collaboration across departments and multiple verification steps, diverting attention from essential tasks. This process not only wastes institutional resources but also places an emotional burden on those involved, affecting morale and productivity. Ironically, these inefficiencies can undermine the very trust these tools aim to uphold.
Ways to Reduce AI Detection False Positives
Reducing false positives in AI detection requires a mix of tools, human oversight, and better AI models. Here are some effective approaches:
Using Multiple Detection Tools
Relying on more than one detection tool can improve accuracy. Each tool has its own algorithm, so cross-checking results helps reduce false positives. For submissions over 300 words, combining analyses from platforms like AI Detector with others increases dependability . Research supports this approach, showing that using multiple methods enhances reliability .
Manual Review of Flagged Content
Human review is essential for catching nuances that automated tools might miss. A clear review process can include:
Component | Focus | Key Checks |
---|---|---|
Contextual Analysis | Writing style consistency | Compare with author's past work |
Language Assessment | Natural language patterns | Look for idioms, nuanced phrasing |
Source Verification | Content originality | Check references and citations |
According to the University of Maryland, human reviewers excel at identifying context and subtleties that AI might overlook . This is especially true for technical or specialized content. While manual reviews address current gaps, future improvements in technology are needed for long-term solutions.
Improving AI Training and Algorithms
Enhancing AI models can significantly reduce false positives. Key steps include:
- Expanding training datasets to include diverse writing styles.
- Regularly updating models with examples of new AI-generated content.
- Developing algorithms that better understand context.
For example, one platform managed to cut sentence-level false positives by 75% after updating its algorithms .
Incorporating Human Review
Structured human oversight adds an extra layer of accuracy to detection processes. Trained reviewers can:
- Apply Contextual Understanding: They assess content within specific contexts, like academic disciplines or industry norms, addressing potential biases in automated systems.
- Provide Detailed Feedback: Instead of penalizing flagged content immediately, reviewers can explain their findings and offer constructive feedback.
- Maintain Quality Standards: Human involvement ensures balanced detection processes, avoiding over-reliance on AI while upholding content integrity.
This combination of tools, human insight, and improved AI models creates a more balanced and effective detection system.
sbb-itb-207a185
Future Developments in AI Detection
Challenges in Reducing False Positives
Despite progress, reducing false positives in AI detection remains a tough challenge as the technology evolves. Three main hurdles stand out:
- Keeping up with the fast-paced advancements in AI-generated content.
- Managing complex natural language patterns that may lead to errors.
- Avoiding bias when dealing with diverse writing styles, especially in technical or specialized fields .
Advances in Detection Technology
In 2023, Stanford researchers reported a 95% accuracy rate using context-aware algorithms, with false positives dropping to just 2% . These results are credited to algorithms that dig deeper into writing context and document structure by focusing on:
- Improved natural language processing.
- Analyzing how well a writing style flows.
- Evaluating the overall structure of documents.
Balancing Accuracy and Fairness
Modern detection systems aim to strike a balance between precision and ethical considerations. This shift addresses earlier issues that unfairly impacted non-native speakers and technical writers . Collaboration between industry and academia is driving the development of systems that are both reliable and fair.
Some key updates include:
- Multi-lingual models that perform well across different languages.
- Transparent systems that clearly explain how decisions are made.
- Adjustable detection thresholds tailored to various writing scenarios.
These strides bring us closer to creating dependable and fair AI detection systems, although achieving flawless accuracy while ensuring fairness continues to be a work in progress.
Conclusion: Key Points
False positives in AI detection are a result of inherent system limitations, impacting both academic and professional settings. For instance, Turnitin's reported 4% sentence-level false positive rate underscores these ongoing challenges.
While advancements like context-aware algorithms (covered in the Future Developments section) have shown progress, such as Stanford researchers achieving 95% accuracy , maintaining this performance across various writing styles and contexts is still a hurdle.
Moving forward, a balanced approach is essential. Relying solely on AI detection tools can lead to serious repercussions, including harm to reputations and wasted resources. A more effective strategy involves combining automated tools with human oversight. This multi-layered verification process ensures better accuracy and fairness, as technology alone cannot address all nuances.
For content creators, AI detectors should be seen as helpful tools rather than definitive judgments. Integrating them into broader verification workflows, alongside continuous technological improvements, can reduce false positives and uphold content quality. The human role in this process remains irreplaceable, as highlighted throughout this discussion.
FAQs
What is the false positive rate for Turnitin?
Turnitin's AI detection tool has a 4% false positive rate at the sentence level, as noted by Chief Product Officer Anne Chechitelli . This means that 4 out of every 100 human-written sentences might be flagged incorrectly. The tool evaluates submissions in two ways:
Analysis Type | Description |
---|---|
Document Level | Reviews the entire submission as a whole. |
Sentence Level | Analyzes individual sentences (4% error rate). |
Short submissions (under 300 words) or those with minimal AI-generated content are more prone to errors. To minimize mistakes, consider verifying results with additional tools, such as those highlighted in the Reduction Strategies section.
"We cannot mitigate the risk of false positives completely given the nature of AI writing and analysis, so, it is important that educators use the AI score to start a meaningful and impactful dialogue with their students in such instances." - Annie Chechitelli, Chief Product Officer, Turnitin