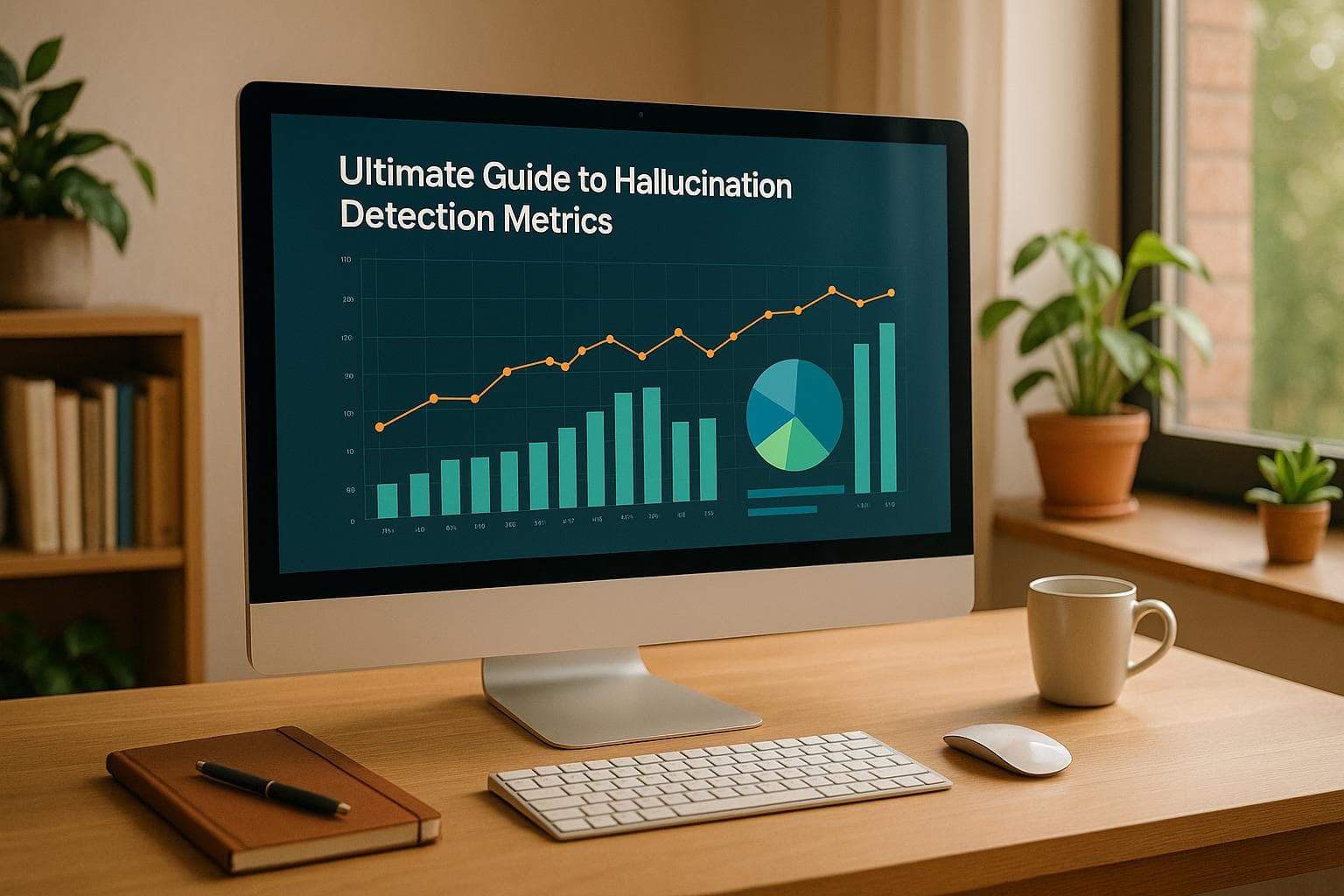
Ultimate Guide to Hallucination Detection Metrics
Hallucination detection metrics help identify false or unsupported information in AI-generated content. These tools are crucial for ensuring accuracy, preventing misinformation, and maintaining trust in AI systems. Here's a quick breakdown:
-
Key Types of Metrics:
- Reference-Based: Compare AI outputs to verified sources (e.g., BLEU, ROUGE, Knowledge-F1).
- Reference-Free: Evaluate reliability without source material (e.g., SelfCheckGPT, SelfCheckGPT-NLI for medical use).
- Hybrid Systems: Combine both methods for higher accuracy (e.g., DeepEval, Kolena Platform).
-
Why They Matter:
- Protects credibility by verifying content accuracy.
- Reduces reputational risks from misinformation.
- Helps industries like education, healthcare, and business ensure content integrity.
-
Performance Insights:
- Tools like AI Detector achieve up to 98% accuracy.
- Advanced systems like DeepEval reach 93.7% F1 scores, processing thousands of samples per hour.
Metric Type | Example Tools | Accuracy | Use Cases |
---|---|---|---|
Reference-Based | BLEU, ROUGE, Knowledge-F1 | 75.4%-82.5% | Academic, publishing |
Reference-Free | SelfCheckGPT, SelfCheckGPT-NLI | 91.7% | Medical, AI content checks |
Hybrid Systems | DeepEval, Kolena | 92%-93.7% | Enterprise, healthcare |
These metrics are evolving to meet diverse needs, from verifying academic papers to authenticating business reviews. They’re becoming essential tools for anyone working with AI-generated content.
Main Detection Metric Types
Hallucination detection metrics are generally categorized into three main types.
Metrics Using Reference Text
These metrics rely on comparing AI outputs with verified source materials to spot discrepancies. Tools like BLEU and ROUGE analyze n-gram overlaps, though their effectiveness varies. For example, BLEU achieves a 75.4% AUC, while ROUGE-L has a precision of 19.2% at 90% recall. A more advanced approach, Knowledge-F1, evaluates claims at the fact level, classifying them as true positives, false positives, or false negatives. It achieves a 0.825 F1 score in hallucination classification by aligning outputs with a knowledge graph.
Metrics Without Reference Text
Reference-free methods assess the reliability of content without needing source material comparisons. One example is SelfCheckGPT, which evaluates consistency across multiple large language model (LLM) outputs, achieving an impressive 91.7% AUC. In medical applications, a variant called SelfCheckGPT-NLI, which integrates the UMLS ontology, improves its F1 score from 0.625 to 0.763 on specialized MedHallu benchmarks. While effective on their own, these methods are often paired with reference-based metrics for better overall performance.
Combined Measurement Systems
Hybrid systems blend reference-based and reference-free approaches for a more comprehensive evaluation. A notable example is DeepEval, which integrates over 14 metrics, including G-Eval and Faithfulness scores, achieving a 0.937 F1 score.
These combined systems are particularly useful in real-world scenarios:
System Type | Accuracy | Processing Speed | Use Case |
---|---|---|---|
DeepEval Ensemble | 93.7% | 10k+ samples/hour | Enterprise RAG systems |
Kolena Platform | 92.1% | HIPAA compliant | Healthcare AI |
Multi-metric (3 metrics) | 93.7% | Cost-effective | FEVER dataset |
For example, the Kolena Platform combines 80% reference-based checks with 20% consistency scoring, achieving 92.1% accuracy in healthcare AI applications. The TrustNLP 2024 guidelines recommend using at least three-metric ensembles with diversity scores above 0.7. This combined approach significantly outperforms single-metric methods, with adversarial testing showing up to a 38% improvement in precision.
Testing Detection Metric Performance
Testing Software Tools
Software tools play a crucial role in verifying the accuracy of hallucination detection. For instance, AI Detector & AI Checker boasts an impressive 98% detection accuracy, as confirmed by rigorous testing. The tool uses advanced algorithms to analyze content across multiple layers while ensuring user privacy. Notably, it does not use uploaded data for AI model training, offering peace of mind to its users. The interface is user-friendly, highlighting AI-generated sentences and providing a percentage breakdown for quick and easy authenticity assessments.
To ensure reliability, these tools rely on standardized test sets for their evaluations.
Test Sets and Measurement Standards
Standardized test sets and protocols are essential for assessing the performance of detection tools across diverse content samples. AI Detector & AI Checker employs thorough verification methods that align with strict academic testing standards. This ensures the tool's credibility and effectiveness in various applications.
Key Success Metrics
The combination of reliable tools, standardized test sets, and clearly defined performance metrics is what drives success in detecting hallucinated content.
"Our AI checker precisely identifies AI-generated content efficiently." - Detecting-AI.com
Key performance indicators include:
- Detection Accuracy: Achieving a 98% success rate in identifying AI-generated content, with real-time processing.
- Processing Speed: Delivering immediate analysis and results.
- Reliability: Consistently performing well across different types of content.
These metrics make the tool valuable for various applications, such as verifying academic integrity, validating content in marketing, and authenticating customer reviews. By meeting these benchmarks, organizations can uphold trust and adhere to established guidelines.
Using Detection Metrics
Setup and System Integration
To ensure accurate detection metrics, it's essential to integrate sentence-level analysis and percentage-based reporting into your existing workflows. These detection tools operate in real-time, offering both pre-publication screening and ongoing monitoring. Striking the right balance between sensitivity and usability is key - this minimizes false positives while maintaining reliable accuracy. With this setup, real-time monitoring becomes a seamless part of your content management process.
Live Monitoring with AI Detector & AI Checker
Once properly integrated, live monitoring takes content verification to the next level. Tools like AI Detector & AI Checker analyze content instantly with an impressive 98% accuracy rate. Some standout features of these tools include:
- Real-time content analysis to catch issues as they occur
- Sentence-level breakdowns for detailed insights
- Color-coded highlights to easily identify AI-generated sections
- Percentage-based authenticity scores for quick evaluations
"Our AI Detector helps you follow university guidelines for essay and paper submissions." - Detecting-AI.com
The system is compatible with a wide range of AI models, such as ChatGPT, Gemini, Jasper, and Claude, and is regularly updated to detect newer models like GPT-4.
Performance Improvement Methods
To maintain effectiveness, the system undergoes continuous updates aimed at improving detection accuracy. These updates ensure the tool can adapt to the latest text-generation trends and techniques.
"Ensure authenticity and protect your reputation." - Detecting-AI.com
Current Limits and Future Progress
Current Metric Limitations
Detection systems have achieved impressive accuracy rates, reaching up to 98%. However, maintaining consistent performance across various types of content remains a hurdle. Each industry has its own unique demands, making it a challenge to refine these metrics to meet the diverse needs of different sectors.
New Detection Methods
Recent breakthroughs have improved both the precision and user-friendliness of detection tools. Modern systems now offer features like color-coded highlights and percentage-based analyses to indicate AI-generated content.
"Maintain trust and avoid the risks of AI-generated content." - Detecting-AI.com
Industry-Specific Uses
With these advancements, detection methods are being tailored to meet the distinct needs of specific industries. Here’s how different sectors are utilizing these tools:
Industry | Primary Use Case | Key Requirements |
---|---|---|
Education | Essay and paper verification | Originality assessment and citation checking |
Research | Source validation | Verification of citations and source materials |
Business | Authenticity analysis | Analysis of customer reviews and online feedback |
Bloggers | Content originality | Plagiarism detection and authenticity checks |
For example, in academic research, ensuring the originality of citations and verifying source materials are critical to preserving scholarly credibility. In the business world, analyzing customer reviews and feedback helps safeguard a company’s reputation.
These advancements and the ability to adapt to industry-specific needs highlight the continuous evolution of hallucination detection tools and metrics.
Summary
Metrics for detecting hallucinations in AI systems have made significant progress. For instance, GPT-4-powered systems now show an 89% correlation with human judgments, a substantial leap from the 62% correlation seen with traditional metrics.
Here’s a quick overview of recent advancements:
Innovation | Performance | Key Benefit |
---|---|---|
Ensemble Methods | 83% F1-score | Combines GPT-4 with semantic analysis |
HHEM Clinical | 70.3% accuracy | Tailored for healthcare content |
Real-time Detection | 90% precision | Uses chain-of-thought flagging for closed domains |
These breakthroughs have paved the way for standardized benchmarks, such as the MedHal dataset. This dataset, with its 50,000 medical examples, enables direct comparisons between systems. For example, newer systems like HuDEx have achieved 89.6% accuracy in question-answering tasks, outperforming GPT-4’s 72.5% accuracy.
Some implementations have delivered standout results, including 92% accuracy in medical documentation and a 45x reduction in detection time.
The growing emphasis on detection systems reflects a broader industry trend, with 40% of AI projects now focused on improving accuracy and efficiency in this area. While advancements have been impressive, challenges remain in achieving consistent performance across diverse domains and content types.
To address these challenges, optimal systems are now integrating LLM-based evaluation with spectral analysis techniques, offering a more robust defense against AI hallucinations.
FAQs
How do hallucination detection metrics enhance the accuracy of AI-generated content in fields like healthcare and education?
The Importance of Hallucination Detection Metrics
Hallucination detection metrics are key to making AI-generated content more reliable by spotting and minimizing instances where the AI produces false or misleading information. This is especially critical in fields like healthcare, where accurate AI outputs can directly impact patient safety and inform crucial decisions. Similarly, in education, these metrics help ensure that learning materials remain trustworthy, preventing the dissemination of incorrect or fabricated information.
By integrating these metrics, industries can rely on AI tools to produce content that is both accurate and dependable, building greater trust in AI-powered solutions.
What are the benefits of using hybrid systems for hallucination detection compared to relying solely on reference-based or reference-free methods?
Hybrid Systems for Hallucination Detection
Hybrid systems bring together the best of both worlds: reference-based and reference-free methods. By combining these approaches, they offer a more balanced and precise way to detect hallucinations in content.
Here’s how it works: reference-based methods cross-check the content against known or verified data, ensuring factual accuracy. On the other hand, reference-free techniques focus on identifying patterns and inconsistencies within the content itself - no external data needed.
This combination not only improves detection accuracy but also reduces the chances of false positives. It’s an especially effective solution in situations where reliable reference data is missing or incomplete, providing a dependable way to evaluate content thoroughly.
What are the main challenges in ensuring hallucination detection metrics remain accurate across various content types and industries?
Challenges in Hallucination Detection Metrics
Detecting hallucinations in AI-generated content isn’t a one-size-fits-all process. One of the biggest hurdles is contextual variability. Different industries and content types have their own standards for what qualifies as a hallucination. For instance, a metric designed to flag inaccuracies in scientific research may not work as well for creative writing or marketing copy, where deviations from strict factuality might be intentional or even desirable.
Another layer of complexity comes from the ever-evolving nature of AI-generated content. As AI models grow more advanced, their outputs become increasingly refined and human-like, making hallucinations tougher to spot. This means detection metrics need frequent updates to stay effective. On top of that, these metrics must account for differences in languages, cultural nuances, and specialized terminology, further complicating the process.