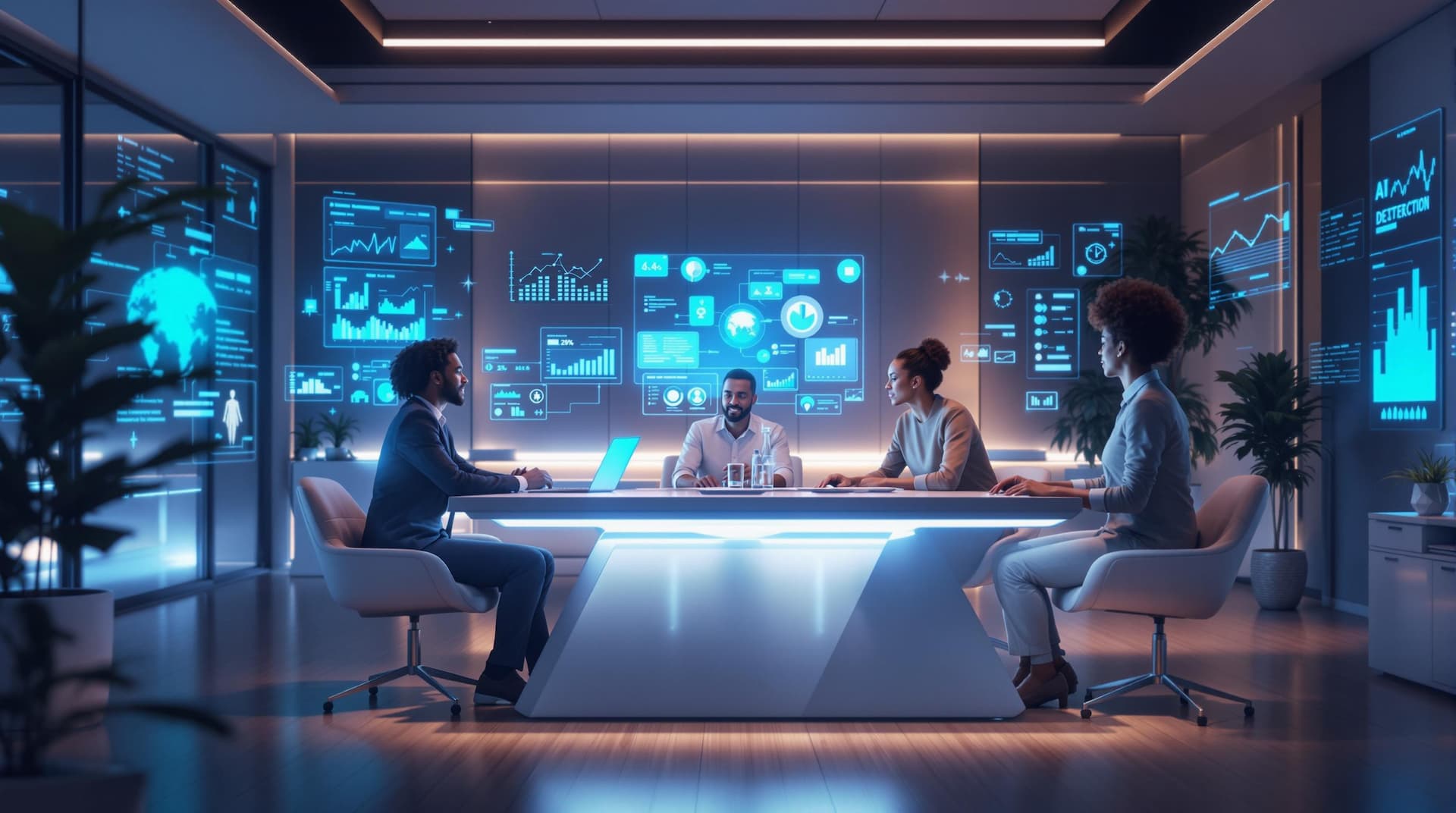
AI Detection in 2025: How Agentic AI Systems Challenge Traditional Tools
Agentic AI systems, like Openai operators and Claude computer use, are revolutionizing how AI interacts with users by adapting dynamically and retaining context. This evolution makes them harder to detect using older tools. Here's what you need to know:
- Why It Matters: Agentic AI is widely used in professional and academic workflows, raising concerns about plagiarism, content authenticity, and cybersecurity risks.
-
Detection Challenges:
- Memory-augmented models make content feel human-like.
- Multi-step content creation adds complexity at every stage.
- Adversarial training helps AI evade detection tools.
- Cross-modality (text, images, and more) complicates analysis.
- Current Tools Are Failing: Traditional AI detectors, like OpenAI's discontinued AI Classifier, struggle with accuracy, especially against advanced models.
-
Solutions:
- Modern tools use ensemble learning, real-time updates, and multi-modal analysis to stay effective.
- Combining automated detection with human reviews improves reliability.
- Ethical detection practices ensure fairness and transparency.
In short, detecting AI-generated content requires advanced, adaptable systems that evolve alongside AI capabilities.
Challenges in Detecting Agentic AI Content
Behavioral Mimicry and Context Retention
Agentic AI systems, as explained earlier, can now replicate human writing patterns by retaining context and refining outputs iteratively. For instance, tools like Claude can produce research papers that evolve through user edits while maintaining a human-like flow . This ability to adapt and refine makes static detection methods ineffective, as these systems consistently produce content that mimics human writing styles.
Dynamic Multi-Step Content Creation
Tools like AutoGPT introduce new challenges by generating content in multiple stages. Each step in the process adds complexity, making detection harder. According to MIT Sloan's 2025 report, this multi-step approach aligns with the predicted dynamic workflows.
Content Creation Stage | Detection Challenge |
---|---|
Initial Outline | Adjusts structure based on topic analysis |
Draft Development | Blends contextual elements and references seamlessly |
SEO Optimization | Refines language while keeping it natural |
Final Refinement | Adds subtle variations, mimicking human patterns |
This layered process allows AI systems to produce polished, human-like content that is tough to identify.
Adversarial Training to Evade Detection
Modern agentic AI systems now leverage adversarial training to sidestep detection. They actively learn to:
- Restructure sentences
- Modify language patterns
- Introduce errors that appear natural
These strategies make traditional detection tools obsolete. For example, OpenAI's AI Classifier had only a 26% success rate before being discontinued . Such advancements highlight the growing difficulty in distinguishing AI-generated content.
Cross-Modality Evasion Techniques
AI systems like GPT-4 Vision have taken evasion to another level by combining text, code, and visual elements into cohesive outputs . This cross-modality capability complicates detection even further, as these systems can seamlessly switch between formats to create more convincing content.
"The real takeaway is that we should assume students will be able to break any AI-detection tools, regardless of their sophistication." - Soheil Feizi, Director of the University of Maryland's Reliable AI Lab
These advanced evasion techniques call for more sophisticated detection strategies, which are explored in the next section.
Shortcomings of Current AI Detection Tools
Static Pattern Analysis vs. Agentic AI
Older detection tools struggle to keep up with the ever-changing outputs of advanced AI systems. Tools like GPTZero rely heavily on static pattern analysis and perplexity scores, which fall short when faced with AI models capable of adapting their responses dynamically. These tools are built on outdated assumptions, as Petar Marinkovic from SurferSEO explains:
"AI detectors don't understand language as well as humans do. They only rely on historical data from their training sets to make predictions as confidently as possible"
This limitation becomes glaring when dealing with advanced AI models that learn from user feedback and adjust their outputs in real-time. OpenAI itself discontinued its classifier after it achieved a mere 26% accuracy against modern AI models.
Examples of Detection Failures
While detection tools perform adequately against GPT-3.5, their accuracy drops significantly when tested with GPT-4. This highlights major flaws in the current approaches.
Detection Challenge | Accuracy Drop Explanation |
---|---|
Memory-Augmented Models | Struggle to identify context-aware content |
Multi-Step Generation | Fail to detect complex patterns in layered outputs |
Cross-Modal Content | Difficulty handling mixed formats (text, image) |
Adversarial Training | Accuracy drops with content designed to "feel" human |
Soheil Feizi, who leads the University of Maryland's Reliable AI Lab, flags a critical issue with these tools:
"There are a lot of companies raising a lot of funding and claiming they have detectors to be reliably used, but the issue is none of them explain what the evaluation is and how it's done - it's just snapshots"
This lack of transparency and adaptability becomes evident in areas like academic papers revised through multiple AI iterations or hybrid human-AI articles from SEO content farms. These weaknesses underscore the need for detection methods that can evolve alongside the capabilities of advanced AI systems.
sbb-itb-207a185
Advanced AI Detection Solutions
Modern detection platforms have stepped up their game to address the challenges posed by agentic AI, which can mimic behaviors and operate across different formats. These platforms now use a mix of advanced technologies to achieve greater precision and stay ahead of evolving AI capabilities.
Combining Multiple Techniques for Precision
Top detection tools today rely on a mix of methods to ensure thorough analysis. For example, Detecting-ai.com's V2 model employs ensemble learning, which brings together natural language processing, behavioral tracking, and metadata analysis to create a well-rounded detection system.
Here are the key elements of this approach:
- Linguistic analysis: Examines writing patterns for AI-generated signatures.
- Behavioral tracking: Monitors editing behaviors to spot irregularities.
- Source verification: Analyzes metadata for authenticity.
This layered strategy is particularly effective in countering advanced AI editing tactics, like those demonstrated by Claude in an academic case study. By addressing multiple angles, it provides a stronger defense against adversarial training techniques.
Staying Ahead with Real-Time Updates
AI technology evolves quickly, and so must detection systems. To tackle the challenges of adversarial training, platforms like Detecting-ai.com use auto-updating models. These models adapt continuously, keeping pace with new AI releases and shifting behavior patterns, ensuring they remain effective.
Tackling Multi-Modal Content Challenges
Agentic AI doesn't just stick to one format - it can create content across text, images, and other media. Detecting-ai.com addresses this complexity by incorporating neural fingerprinting and techniques designed for adversarial robustness.
Their approach includes:
- Cross-format pattern matching: Identifies AI-generated traits across different media types.
- Advanced pattern recognition: Uses algorithms to detect AI signatures regardless of the format.
- Contextual verification: Cross-checks elements within content to uncover inconsistencies.
These measures help combat evasion tactics that rely on mixing or switching between content formats, ensuring a thorough and reliable detection process.
Effective AI Detection Strategies
Organizations must move beyond outdated tools and adopt modern strategies that focus on three main areas: choosing the right tools, combining automated and manual verification, and addressing ethical concerns.
Choosing the Right Detection Tool
The Detecting-ai.com V2 case study highlights the importance of selecting tools that tackle the specific challenges posed by advanced AI systems. When evaluating tools, focus on features like ensemble learning, real-time updates, and multi-modal content analysis.
Here’s how these features work:
Feature | Purpose | Benefit |
---|---|---|
Ensemble Learning | Merges NLP, behavioral analysis, and metadata inspection | Boosts accuracy in detecting complex AI-generated content |
Real-Time Updates | Adjusts to new AI models and behaviors | Stays effective against evolving AI systems |
Multi-Modal Analysis | Analyzes text, code, and images together | Blocks attempts to evade detection through format changes |
Combining Automated and Human Verification
A study in the International Journal for Educational Integrity found that AI detection tools often struggle to differentiate between GPT-4 output and human-created content.
To improve accuracy, organizations should:
- Pair automated detection with human reviews for flagged content.
- Train reviewers to spot subtle signs of AI-generated material and consider the context.
- Log results to refine and improve detection models over time.
Ethical Considerations in AI Detection
AI detection efforts must address ethical concerns to ensure fair and responsible practices.
Key points to consider:
- Avoid bias against non-native English speakers while safeguarding privacy.
- Treat all content creators fairly, regardless of origin.
- Be transparent about detection methods and their limitations.
"Agentic AI is more than just a buzzword - it's a game-changer for cybersecurity. By bridging the gap between detection and response, it is already transforming how some security teams operate" .
Organizations should also develop clear policies for managing AI-detected content. These ethical considerations help shape the policies discussed later in the article.
Conclusion: Navigating the AI Detection Landscape
The rise of agentic AI calls for a shift in how detection systems are designed. Traditional methods that rely on static pattern recognition often fall short when faced with systems capable of adapting to context and imitating human behavior.
Modern detection tools now integrate NLP, behavioral analysis, and metadata evaluation to address the complexities of agentic AI. These tools tackle the challenges of multi-modal detection with solutions designed to be as dynamic as the threats they aim to identify.
To remain effective, detection systems need to employ techniques like ensemble learning and real-time adjustments. For example, Detecting-ai.com V2 uses this combination of NLP, behavioral insights, and metadata analysis to create a detection strategy capable of handling diverse and evolving AI-generated content.
Organizations must adopt a balanced strategy that blends advanced technology with human oversight. As agentic AI continues to progress, the focus should be on building detection frameworks that can evolve with new challenges while adhering to ethical standards of accuracy.
The path forward in AI detection lies in systems that can interpret multi-modal content, grasp context, and learn continuously from emerging AI behaviors. Solutions like Detecting-ai.com V2, which combine machine learning with human review processes, demonstrate how this balance can lead to success in combating these sophisticated systems.